
‘We Are Just Scratching The Surface': Expert On The Potential Of AI And Future Of Work
Author and Academician Arun Sundararajan discusses the transformative potential and challenges of AI in an interview with Govindraj Ethiraj at The Core.
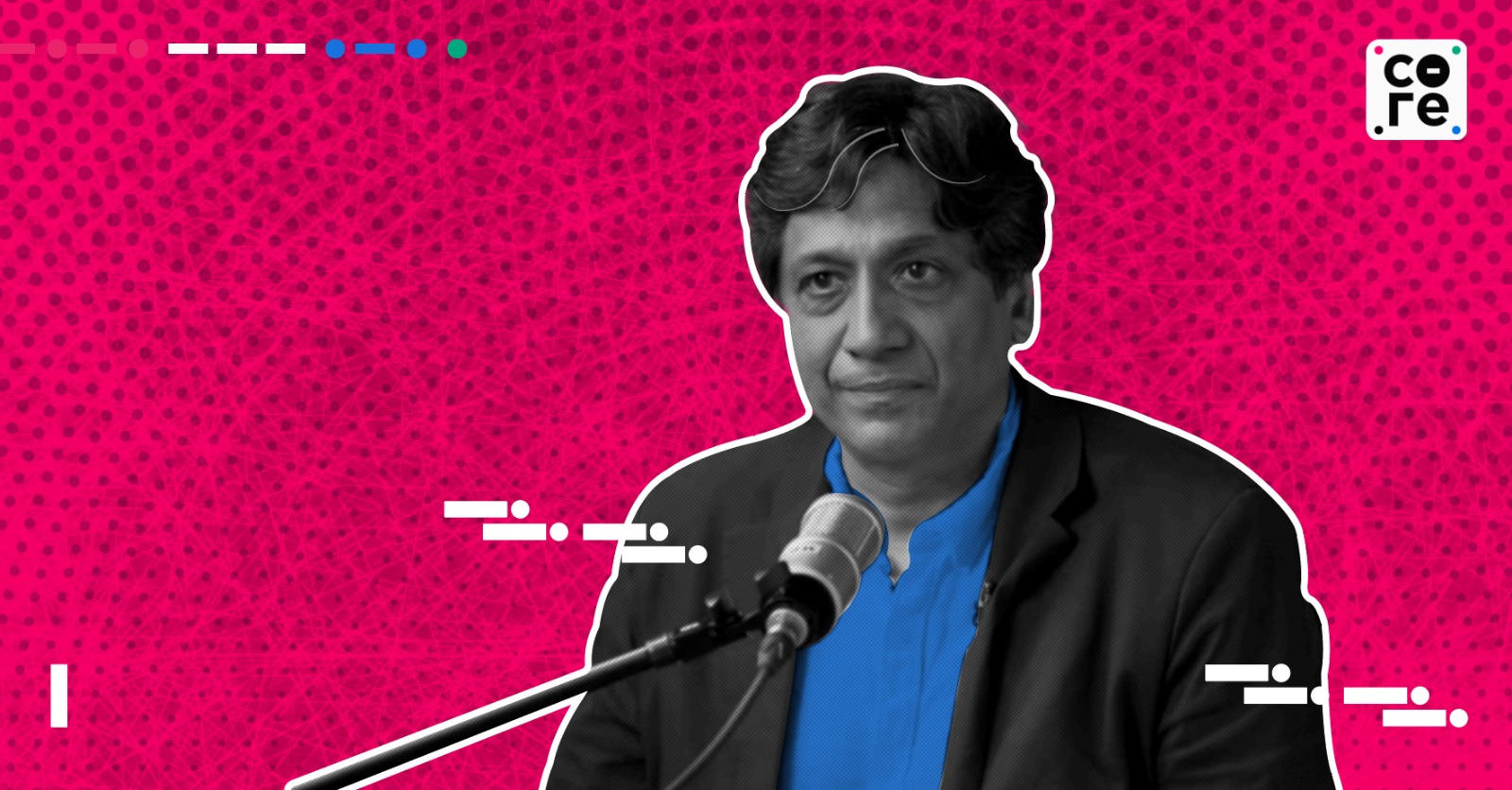
OpenAI's ChatGPT has everyone worried about one thing in particular - the future of work and if more people will be out of jobs as Artificial Intelligence (AI) becomes better and better.�
AI has seen resounding success since the launch of ChatGPT in 2022. But one of the major concerns is how it could spread disinformation. Even Sam Altman, the CEO of OpenAI is worried about it. He is also worried about how AI could be used in cyber attacks as it is adept at writing code.�
While there are apprehensions about AI taking over work culture, it is also being seen as a tool to revolutionalise sectors like health and education which is often seen to be suffering due to the lack of manpower.� �
The Core's founder and financial journalist Govindraj Ethiraj spoke to author and academician Arun Sundararajan on how AI could change the future of work and risks of disinformation that could come with it.�
"Healthcare is an exciting example where you can dramatically expand the reach of particular kinds of non-critical healthcare to areas where you may not have a qualified doctor or you may not have a person who is qualified to elicit symptoms and th...
OpenAI's ChatGPT has everyone worried about one thing in particular - the future of work and if more people will be out of jobs as Artificial Intelligence (AI) becomes better and better.
AI has seen resounding success since the launch of ChatGPT in 2022. But one of the major concerns is how it could spread disinformation. Even Sam Altman, the CEO of OpenAI is worried about it. He is also worried about how AI could be used in cyber attacks as it is adept at writing code.
While there are apprehensions about AI taking over work culture, it is also being seen as a tool to revolutionalise sectors like health and education which is often seen to be suffering due to the lack of manpower.
The Core's founder and financial journalist Govindraj Ethiraj spoke to author and academician Arun Sundararajan on how AI could change the future of work and risks of disinformation that could come with it.
"Healthcare is an exciting example where you can dramatically expand the reach of particular kinds of non-critical healthcare to areas where you may not have a qualified doctor or you may not have a person who is qualified to elicit symptoms and then give an opinion," Arun Sundararajan told The Core.
Here are the edited excerpts from the interview:
Govindraj Ethiraj: Talking about the present of AI first, we are currently still at this point where we are awestruck by what we are seeing around us. Not just ChatGPT itself or regenerative AI but also the way people are finding interesting applications. And everyday there's a firm or maybe many firms using ChatGPT API to create something interesting of their own. So tell us about how you are seeing this evolve.
Arun Sundararajan: I am excited by the emergence of Generative AI and I think it has been a long journey in many ways back from the expert systems of the 1990s to the rise of machine learning since the mid-1990s. Then the coming-of-age of finding your own features with neural networks where you did not have to rely on the humans to say this is what matters. And now to the generation of these large language models that are able to generate content on their own. They are able to generate images on their own. I think one of the things that is most compelling about these large language models is the way in which you can relatively easily customise them with a few examples to do something new. It is like having an incredibly smart person who has a real breadth of general knowledge and who knows all of the rules of English and so on. And if you just give them a few instances of something new-sort of here is how to rank reviews–here is a bunch of conversations with my customers, here is a bunch of specialised information about my particular line of business. They then use the general knowledge and the language knowledge that they have to suddenly become an expert in what you want them to become an expert in. So I think we are in a very early stage. We are just scratching the surface in terms of what the potential of these large language models are going to be for specialised business applications.
Govindraj Ethiraj: That is interesting because it is also a very frightening description. What you are saying is that someone who is essentially a master of none suddenly becomes an expert in something.
Arun Sundararajan: That is true and we have not yet tested the depth of this expertise but you know we could create for example a digital twin of Govind. There is plenty of content out there that describes how you think, how you write, how you speak and so on. So, it would not take too long to fine tune a GPT model to start to generate content like you. Now the open question is how far can it go in terms of matching the human Govind. That is something that is an i/empirical question that we are going to have to see the answer to over time.
Govindraj Ethiraj: You use this example because you feel that people are already working on this?
Arun Sundararajan: They have worked on it already. There are some good examples. I mean, like the Khan Academy has a teaching assistant, Khanmigo which they spent a lot of time fine-tuning in collaboration with Microsoft. It does a better job than any digital teaching assistant that I have encountered in being able to not give you the answers but teach you how to understand something. And again this was sort of fine-tuned to the specific application of education.
I am seeing examples in a wide variety of other domains as well. I think healthcare is an exciting example where you can dramatically expand the reach of particular kinds of non-critical healthcare to areas where you may not have a qualified doctor or you may not have a person who is qualified to elicit symptoms and then give an opinion. So, the sky's the limit at this point.
Govindraj Ethiraj: Do you also see similar applications in financial systems or financial planning?
Arun Sundararajan: Absolutely. I think one of the areas where there is a very huge market potential is in sort of mid-range financial advice. Part of the reason for that is that the set of people who have a dedicated human financial advisor is presently much smaller than the set of people who could benefit from a dedicated human financial advisor but ,you know, just can't afford one. From the financial advisors point of view there is no real business model for them to answer a few questions from a lot of people. Hence, they tend to build deep relationships with a small number of high net worth or sort of mid high range net worth people. So in that space, I think, LLM-based (large language model) financial advisors are going to take off once we figure out how we can train them in a way that elicits sufficient trust from a person who is seeking that financial advice.
I mean one of the interesting things that has come up on the trust front is-at least in the U.S it is much easier to get someone to trust an AI-based system for healthcare advice than it is to get them to trust an AI-based system for financial advice. Because it really has to do with what is the human equivalent-again in the U.S your typical experience with a doctor is largely someone you do not know, wearing a white coat; .and you trust… They say they are doctors. They tell you what is wrong with you and you trust them. So the AI experience is not that far away.
On the other hand in financial advice-for a lot of people-there is no sort of analogous human experience. For the few people who{do} have a dedicated financial advisor, the trust was built over years. And so just listening to a BOT… after a few minutes of interaction does not ramp up trust to the level that is necessary to make consequential decisions.
Govindraj Ethiraj: As you were talking about AI making us lose the intellectual property rights over our own intelligence at a Tedx Gateway talk. Tell us about that.
Arun Sundararajan: That is true. It is an issue that is not widely discussed but which I am increasingly concerned about because of the ease with which these new generative AI systems can be customised to do a specific thing. That specific thing can be to mimic the creative behaviour of a particular individual.
We have already seen it happen in the music and art domains where you can pretty much pick any band and can train a generative AI model to create new music in the style of that band that is indistinguishable from what the humans have created. It is the same thing with cartoonists.You can create
Govindraj Ethiraj: And we are seeing that already all over social media.
Arun Sundararajan: So in some ways you are taking away someone's ability to own how they create. And I guess tomorrow this will come to the corporate sector where maybe you will start by saying let us train a large language model to be my digital twin to offload some work. It can draft memos in my voice, it can reply to email, etc.However, there is no guarantee that this digital twin is not going to stay behind if you switch employers. And that is the issue that I am trying to raise awareness about and come up with a solution for. Which is that as things currently stand we have not really thought about- what are our intellectual property rights over our creative process. It has all been around specific instances of what we create. And this is not the fault of the lawyers or the legislators. They never really had to think about--do you own your intelligence;it was by default of course you do. So I think the solution really lies in extending, in some ways the intellectual property infrastructure to cover if something is trained specifically to replicate parts of a human being then the human being should have ownership rights over that replica and some kind of joint ownership claim over what that replica creates. So if your AI generates new content you should have some kind of ownership rights over it-the way you have ownership rights over what you generate.
Govindraj Ethiraj: Why are some tech companies already soliciting legislation? Is that because they are trying to catch up and they want time to catch up or because they are genuinely worried about what this can do?
Arun Sundararajan: I think it is a mix of different reasons. I think everybody who has thought about AI policy realises that there is no comprehensive way in which technology can be the solution. Take the simple example of creating deep fake videos. That technology is out there. I mean saying that the technology companies have to prevent this from happening technologically is simply not possible anymore. The only solution is legislative-to say that creating deep fakes is illegal in some way,misuse of your likeness is illegal, misuse of your voice is illegal, creating a digital twin of yours without your consent-some of these things simply have to be illegal. I think what that is going to require is sort of striking the right balance between openness and chasing innovation and some sort of licensing and control.
One thing that I think is a good idea is to at least in the early stages, if you classify a particular AI as having sufficient risk to only allow people who have licensed infrastructure to be able to run that kind of AI commercially. Some people are calling this-Know Your Cloud, sort of a new kind of KYC.
So in the past when looking at tech-related regulatory questions. My initial instinct has always been to go down the self-regulation path and then wrap the government around where we see market failure. But this is an exception. This is a case where it is very clear that legislation has to lead the way.
Are the tech companies asking for legislation because they genuinely want the problem solved? I think it is partly because they realise the solution has to be a combination. I think it is also in part because it allows you to…if you are a large tech company. Having a robust regulatory infrastructure early on may preserve your leadership position at a time when a lot of this AI is going open source. And there is a worry about how am I going to retain any of the value that I am creating? I think it is less about catch-up. Google and Microsoft are leaders in different ways and Microsoft and Open AI have won the initial chatbot war but Google has perhaps the deepest bench of AI talent in the world. They may not release it as fast because it might interfere a little with their search business and they have got a different kind of balancing act to strike.
But these are both companies in leadership positions and they are both calling for regulation. So I think it's a combination of wanting to make sure that AI suddenly is not banned completely because terrible things happen and wanting to make sure that their leadership position is preserved to some extent and not constantly threatened by open source and small companies.
Govindraj Ethiraj: You said terrible things happen and disinformation comes to mind. Sam Altman himself is on record to say that this is one of the biggest downsides of ChatGPT as he sees it. So where will this go and how could this go as things stand in your own understanding?
Arun Sundararajan: Well a couple of things could happen.One is we may start to rely more and more on content that is generated by an AI as our initial point of generating content itself. We may start to believe that the answers that are coming from large language models are the right answers. People often ask me: why does ChatGPT get things wrong? Why does it make up things? And part of my answer is: You have to realise that ChatGPT is making up everything that it says. Every word is made up. The surprising part is so much of it is actually correct and true. If we do not get a good regulatory system around this early, then we of course run the risk of rampant misinformation, potentially hate speech. We also have to pay very close attention to the kind of bias that is embedded in these large language models because,again, they are not programmed in a particular way. They are learning from examples. So they may have a particular cultural orientation that may not represent the global context in which they are applied. They certainly have some traditional sort of patriarchal bias in them
Govindraj Ethiraj: Patriarchal bias, liberal bias?
Arun Sundararajan: Yeah, so if you ask about scientific discoveries they are more likely to make up the name of a man rather than a woman. And if you ask about company founders-I've tried all this. You make up the name of a company and ask who founded this company, it will give you the name of an Indian man. So the particular cultural and patriarchal bias that ChatGPT has. These are some of the early dangers. I think in the long run it really has to do with loss of control over our intelligence. At some point you will reach a place where I do not believe that AI can generate all of its knowledge itself. I mean you have to inject human-created knowledge into the process but we should not reach a point where there's no incentive for a human being to do this.
Govindraj Ethiraj: India has manpower intensive jobs and businesses. IT, services, exports and so on. The value addition is lower. And therefore the fear that AI in many forms could take that over.
Arun Sundararajan: It is a little early to make bold predictions about exactly where this new type of AI is going to displace jobs. But early indications are that these large language models are very good at a few things-they are good at coming up with first drafts, so initial content ideation and generation. They are fairly good at content summarisation. They are good at structured conversation, even specialised conversations. So if you give a large language model enough examples that pertain to technical support for a particular television it can learn how to provide technical support for this television relatively fast. They are also good at creating simple computer programs. So as the complexity grows, I think they are still limited. If you think about jobs that involve understanding the syntax of a language and generating simple computer programs that other more expert programmers than assemble. If you look at customer support and if you look at sort of the less thoughtful branches of journalism that are generating clickbait, for example. Anyone who is in any of these professions is facing a serious and immediate threat from large language models.
I think certainly on the customer support line, on the customer support side and on the sort of the less complex computer programming side-both of these could hit India because there are sort of large fractions of the global workforce in each of these areas that do sit in India.
Govindraj Ethiraj: In India there's been a lot of emphasis on learning coding early and maybe elsewhere too in the world. How do you see that? Do you still feel it is necessary…should we be now thinking of something else rather than just coding particularly young people.
Arun Sundararajan: I still think it's a really good idea to teach people computer programming early on and part of the reason is: the same reason why it is good to teach them Math.It wires your brain in a way that is good like you are sort of learning certain ways of thinking and ways of problem solving through the programming. I think the emphasis should be more on concepts and less on syntax. So I don't think there's much of a return from being the expert who knows the python command solve a particular problem… but understanding recursion, understanding these concepts I think that's still valuable because you know we're not going out into the job and doing differential calculus right but we are benefiting from having learned that in middle school or high school right.
Govindraj Ethiraj: How should organisations be looking at this… I am talking about the opportunity first and then perhaps the threat.
Arun Sundararajan:Well, I mean, certainly one area where organisations could definitely benefit is in knowledge management-that's a place where every organization should be looking. You know this has been in the lingo since the early days in the internet. The early web dream was that because of the web we will now be able to access all of the knowledge in our organisations, search for it through like a customised search engine. And the results have been mixed. But now with the possibility of being able to take like the knowledge that is sort of embedded in ... .like unstructured text and train something to understand it in its own way and be a resource for people in your company… I think there's a huge opportunity in that . Whether you are from the pharma sector or whether you are creating specialised chemicals for paints or whether you're in financial services or legal services… Knowledge Management is certainly a priority
Govindraj Ethiraj: If there is a digital twin then could you leave the company and your digital twin could continue to run operations?
Arun Sundararajan: I think up to a point. Over there is sort of a balancing act between like you know how much. Maybe it's not that different from when in some organisations/occasions you delegate to an employee you are always sort of striking a balance between how much should I give up and how much should I keep to myself ? How much should I preserve my own position? So you are going to probably face an equivalent thing with your digital twin but we are in a much less understood space. I think for organisations definitely looking at customer engagement and interaction that's definitely a space where AI has so far failed to really deliver until these large language models came along. We have all faced the frustrations of shouting at these voice response systems over the phone and then we look at Siri. Siri seems to understand what we say and we wonder.. and the answer is because Siri is customised to you. Now once we enter this area where a large language model where there is enough technology to parse what you are saying and converted into text and the large language models are trained on specialised areas both on the sales point of view and the customer support point of view. There is definitely low hanging fruit for most organisations.
Govindraj Ethiraj: Today you're focused on AI and obviously this is an important phase and perhaps more critical and important at the same time. How are you spending your own time trying to keep up with this?
Arun Sundararajan: I think most of us are playing a battle of catch-up where I'm fortunate. I was programming as a kid. I built expert systems when I was in college and so I'm starting with an advantage relative to most business professors because I have some sort of background in computer science but even I've had to spend a few hours a day just trying to figure out exactly what the technology capabilities are. So on the AI front I think that that has slowed a little. You have to be willing to invest a couple of months in getting up to speed with how these things work at least at a high conceptual level. In terms of other things that I'm thinking about outside of AI, I've been spending a lot of time thinking about this move towards digital sovereignty. There are countries that are not just on the privacy front but on other fronts. There seems to be a trend towards wanting national self-sufficiency in the creation of digital of different kinds. You have seen the US moving towards wanting to become more self-sufficient on the semiconductor and chips front.
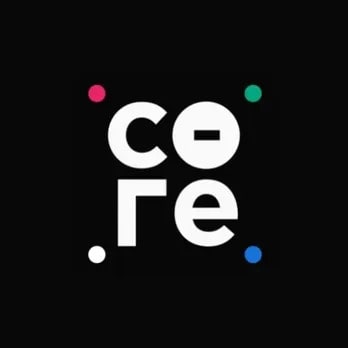
Author and Academician Arun Sundararajan discusses the transformative potential and challenges of AI in an interview with Govindraj Ethiraj at The Core.